
Governance Resources
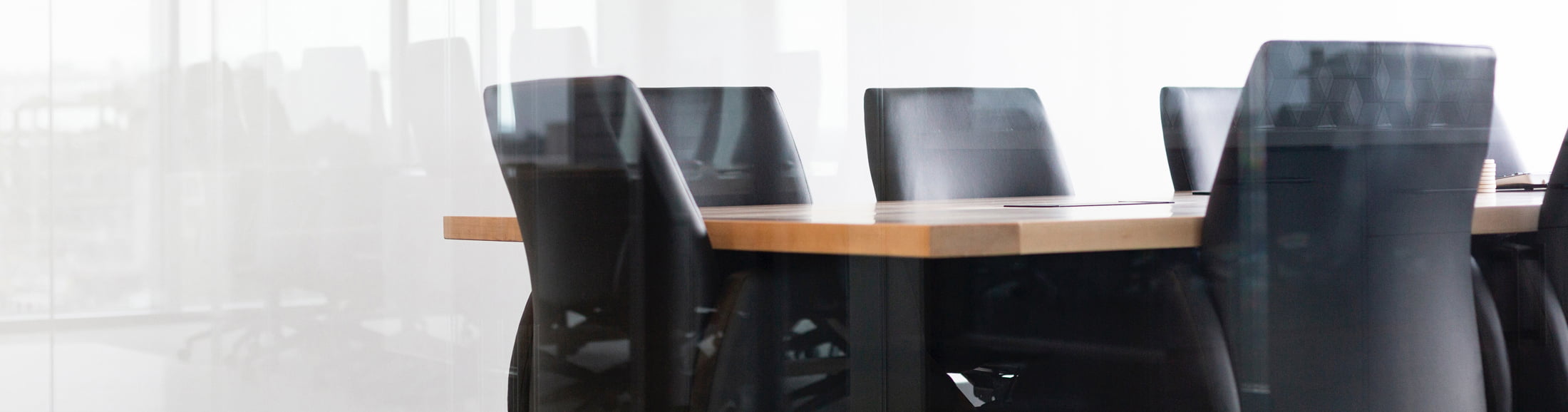
Trending Oversight Topics
Governance Surveys
Center for Inclusive Governance

Elevating the Board’s Oversight of Human Capital
Unlock potential using these resources to oversee your company’s human capital management more broadly.

Recently Seen in Directors Daily®
Explore featured content from your favorite email newsletter.

NACD Blue Ribbon Commission Report
Culture as the Foundation:
Building a High-Performance Board
The 2023 Blue Ribbon Commission report calls on boards to explicitly use culture as an instrument to drive board excellence in today’s complex environment.

Featured Report
2023 Public Company Board Practices and Oversight Survey
The latest boardroom trends and activities for directors of publicly traded companies.

Explore by Governance Topic

Explore the New NACD Search
Search By Title, Author, Topic, and More

Online Articles from Directorship®
The most up-to-date and noteworthy articles including our former BoardTalk blog.

Recommended Courses & Events
Discover More
NACD Directors SummitTM